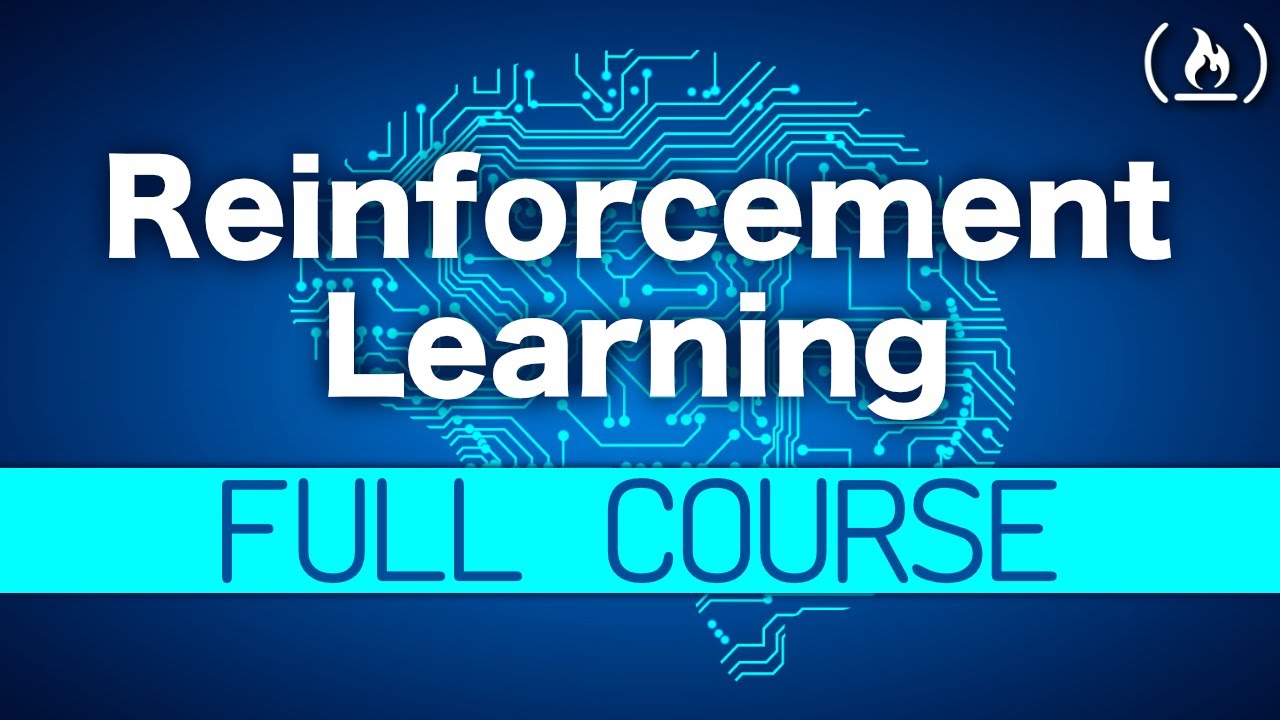
The course covers Q learning, SARSA, double Q learning, deep Q learning, and policy gradient methods. These algorithms are employed in a number of environments from the open AI gym, including space invaders, breakout, and others. The deep learning portion uses Tensorflow and PyTorch.
The course begins with more modern algorithms, such as deep q learning and policy gradient methods, and demonstrates the power of reinforcement learning.
Then the course teaches some of the fundamental concepts that power all reinforcement learning algorithms. These are illustrated by coding up some algorithms that predate deep learning, but are still foundational to the cutting edge. These are studied in some of the more traditional environments from the OpenAI gym, like the cart pole problem.
π»Code:
⭐️ Course Contents ⭐️
⌨️ (00:00:00) Intro
⌨️ (00:01:30) Intro to Deep Q Learning
⌨️ (00:08:56) How to Code Deep Q Learning in Tensorflow
⌨️ (00:52:03) Deep Q Learning with Pytorch Part 1: The Q Network
⌨️ (01:06:21) Deep Q Learning with Pytorch part 2: Coding the Agent
⌨️ (01:28:54) Deep Q Learning with Pytorch part
⌨️ (01:46:39) Intro to Policy Gradients 3: Coding the main loop
⌨️ (01:55:01) How to Beat Lunar Lander with Policy Gradients
⌨️ (02:21:32) How to Beat Space Invaders with Policy Gradients
⌨️ (02:34:41) How to Create Your Own Reinforcement Learning Environment Part 1
⌨️ (02:55:39) How to Create Your Own Reinforcement Learning Environment Part 2
⌨️ (03:08:20) Fundamentals of Reinforcement Learning
⌨️ (03:17:09) Markov Decision Processes
⌨️ (03:23:02) The Explore Exploit Dilemma
⌨️ (03:29:19) Reinforcement Learning in the Open AI Gym: SARSA
⌨️ (03:39:56) Reinforcement Learning in the Open AI Gym: Double Q Learning
⌨️ (03:54:07) Conclusion
Course from Machine Learning with Phil. Check out his YouTube channel:
--
Learn to code for free and get a developer job:
Read hundreds of articles on programming:
And subscribe for new videos on technology every day:
0 Comments